Phytologist
Phytologist generates an annually growing 3D tree geometry to represent billions of unique individuals for a specific species.
Phytologist, A Tree Nursery at Your Fingertips
By utilizing highly specialized DirectX 12 shaders, the Phytologist program can generate billions of unique 3D tree models that are both crisp and accurate. With 77 currently available species to choose from, you can have billions of unique and individual tree models that are highly detailed from the foliage to the bark.
Life-Like 3D Tree Models
Following realistic growth patterns based on the species of tree, Phytologist makes it easy to track and view the growth and development of a tree from the very first year until the end of its life. Using real-world characteristics from individual species, Phytologist uses things such as tree form, branching, foliage, and canopy shape to generate accurate and life-like 3D tree models. Canopy shape is honored differently by each species as some species only have a rough canopy shape, others sharply honored.
Highly Detailed Foliage and Growth
As trees grow, they can become varied, and not all look the same. Phytologist takes this into account when generating your tree models. Allowing tree forms to become truly unique and individual as they grow and differ from a young age throughout their maturity. Things such as foliage budding are determined annually, allowing even the same species of trees to become one-of-a-kind. Most tree species can be identified by their distinctive foliage, Phytologist faithfully recreates this species-specific foliage in high detail.
Realistic Variety
A tree's growth can be altered by things such as annual precipitation patterns or dying branches, leaving the tree unsymmetrical as it grows. By taking this into account, Phytologist is capable of rendering trees based on water patterns or things such as dead or fallen branches that could alter the appearance or hinder growth.
Putting Phytologist to Work
Phytologist is just the first complicated and necessary step to being able to provide a generative AI to allow the inclusion of trees in AI training data. To train a machine learning model to a productive and useful level millions of labeled training images are required. Collecting real-world images is in many cases nearly impossible, labeling then takes a large time-consuming effort. Reaching the level of millions can be prohibitively expensive.
A recent development to be able to solve this problem is the use of realistic 3D models to generate training images. The models themselves contain the information to also be able to generate a matching labeling image. Using this training data generation technique, it is possible to represent any scenario required for AI model training, but the outdoor world is complicated.
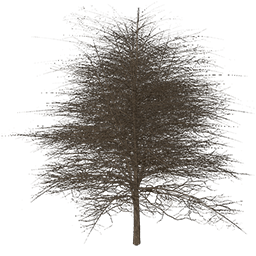
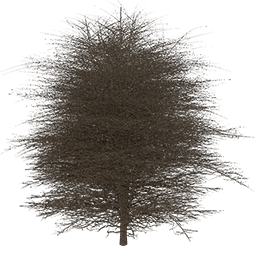
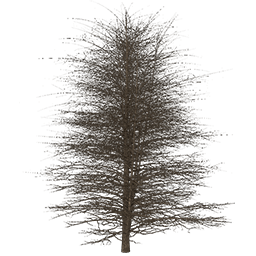
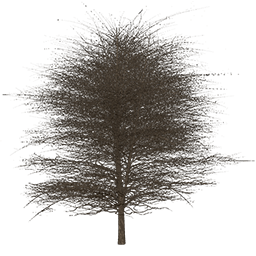
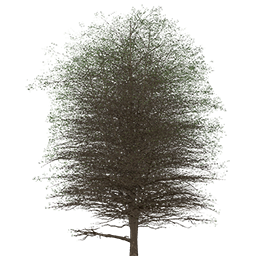
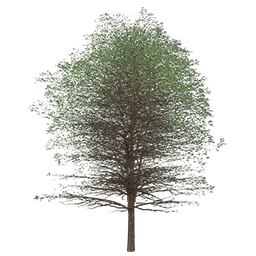
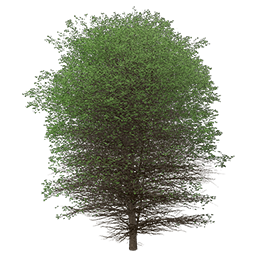
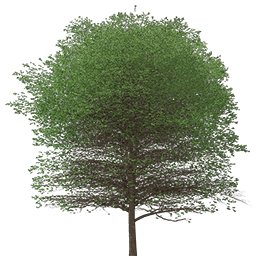
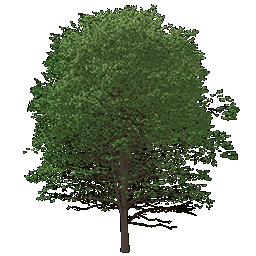
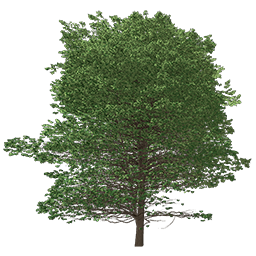
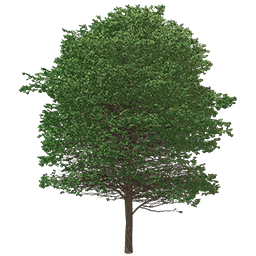
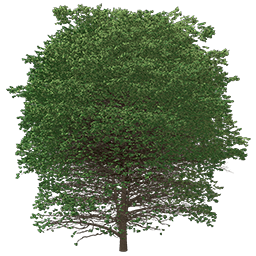
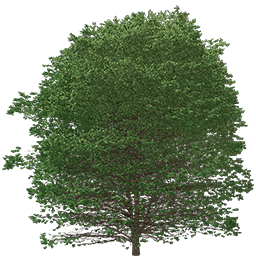
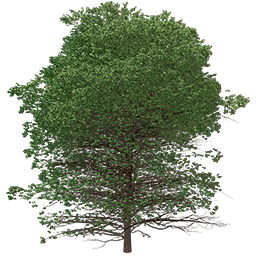
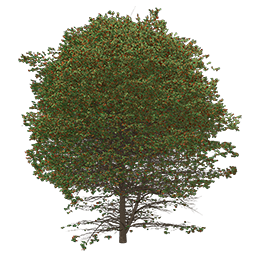
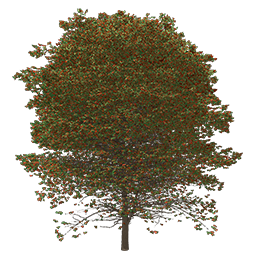
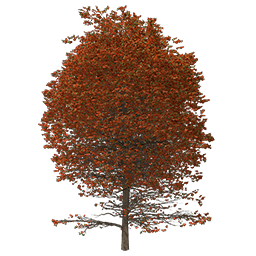
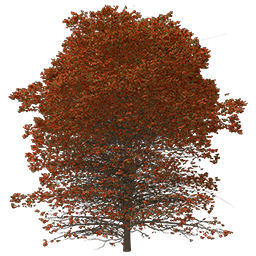
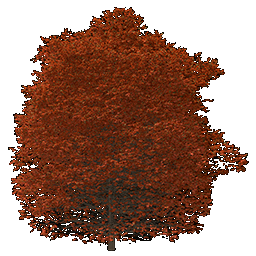
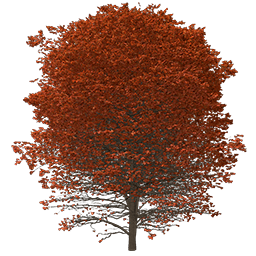
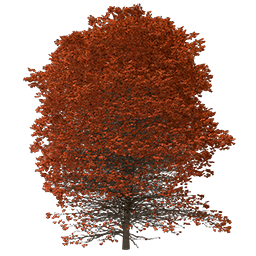
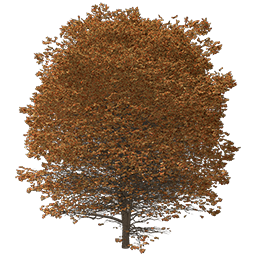
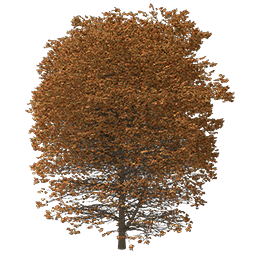
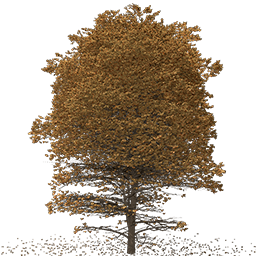
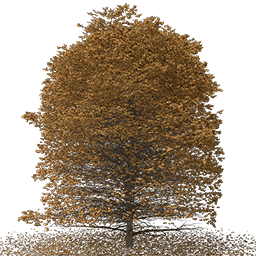
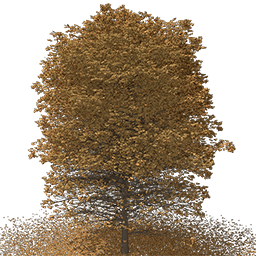
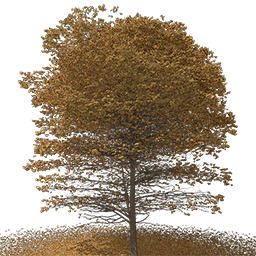
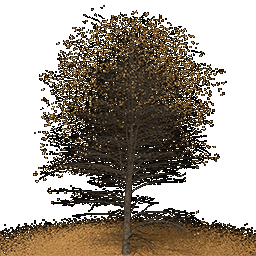
28 unique 50 year-old Silver Maples in different seasons for training.
Two AI Models Needed
Next steps are to use the image generation of millions of each species over their life span with seasons to be able to train a machine learning classifier to be able to recognize a specific species. At the same time generate tens of millions of training images from unique individuals for a single species. Using these training images to start training a generative AI model with the goal of being able recreate a specific image of that species.
The classifier model can then be used to cross check the generative AI model output. Then on to training the generative AI on the next species. The end goal is to be able to use the generative AI model to be able to add realistic trees to any 3D model training data complete with the tree labeled. But why is this needed?
AI Models that Operate in the Real World
Trees can present a hazard to power lines, roads and rail lines. When a hurricane makes landfall hundreds of square miles are impacted by the hurricane force winds. After the hurricane has passed, understanding the impact on the power and transportation infrastructure is a slow and time-consuming process.
Drones can easily be deployed to survey and provide a video feed of the affected areas, but it takes a large effort to identify the hazards, an AI model properly trained could do this in near real time. Matching the GPS position of the drone with the video frame of an identified hazard or potential hazard would allow for the rapid development of a response task list to clear the infrastructure.
Drones will fly above tree level and normally have an oblique view of their world. Since these trees are fully 3D this is not a problem and the training for use in drones can be optimized by using oblique tree images.
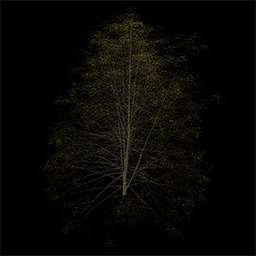
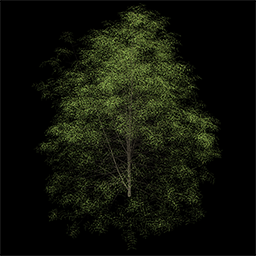
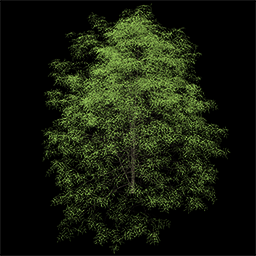
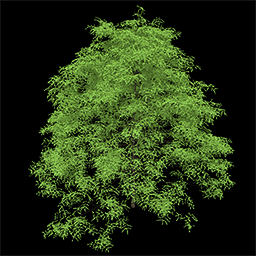
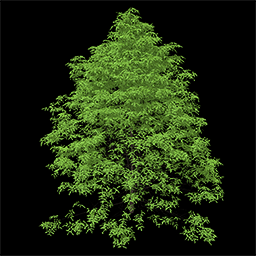
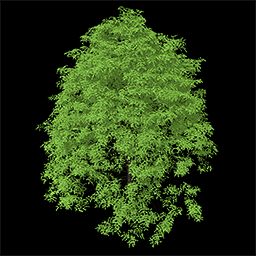
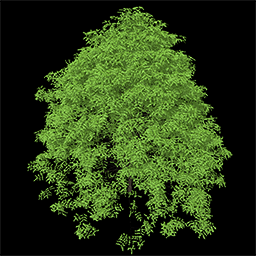
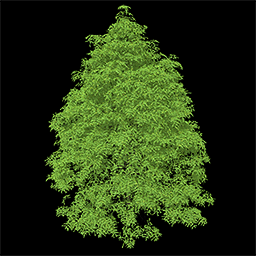
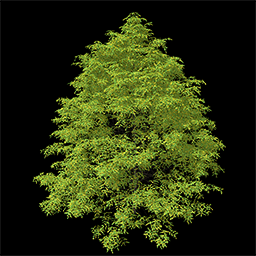
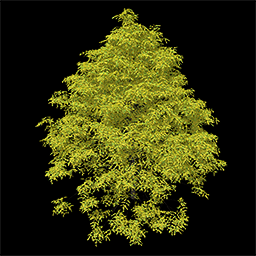
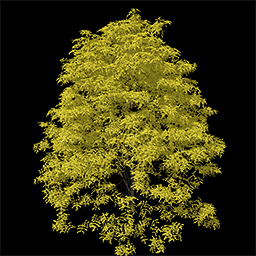
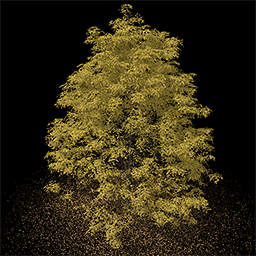
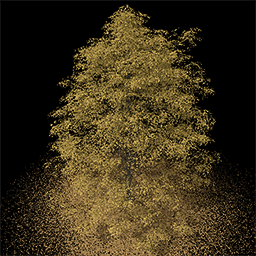
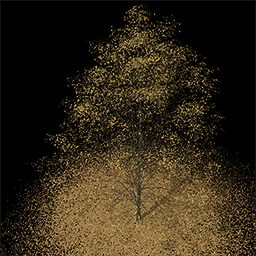
14 unique 60 year-old Kentucky Coffee Trees using an oblique view with different seasons for training.
If AI models need to work with aerial camera data the training may be best done using top views. Although resolving specific species may be very difficult unless the aerial imagery is very high resolution.
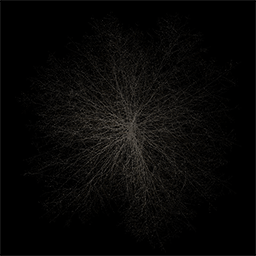
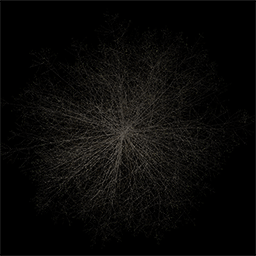
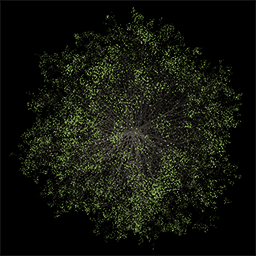
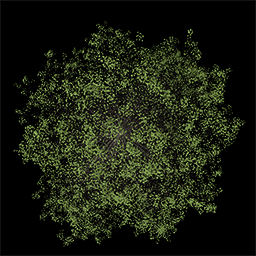
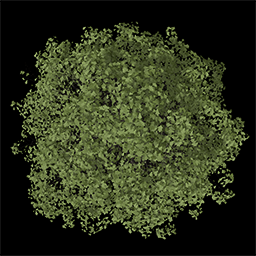
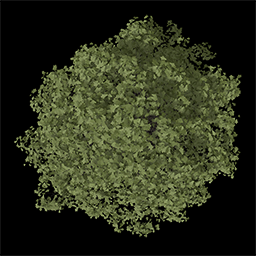
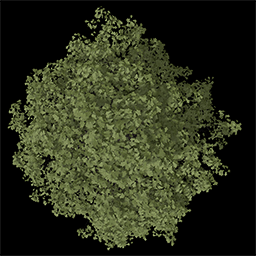
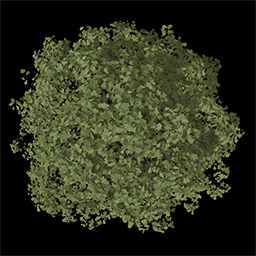
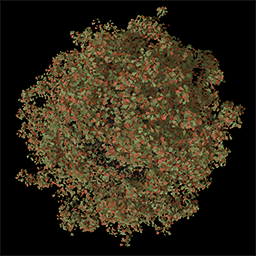
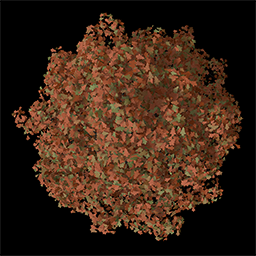
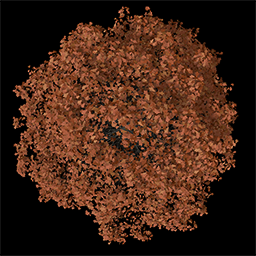
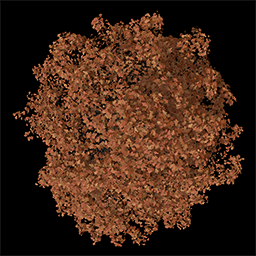
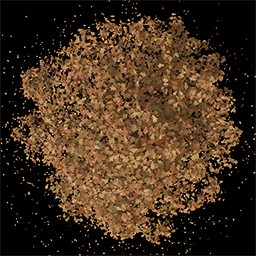
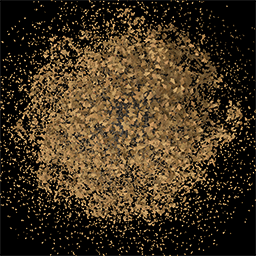
14 unique 80 year-old Northern Red Oaks using top view with different seasons for training.
Technology Demos
5 unique Kentucky Coffee Trees growing over the first 28 years.
Seasonal changes over a year.
3D Eastern Redbud trees rendered with wind.
3D fall Silver Maples with wind.
Tree Samples
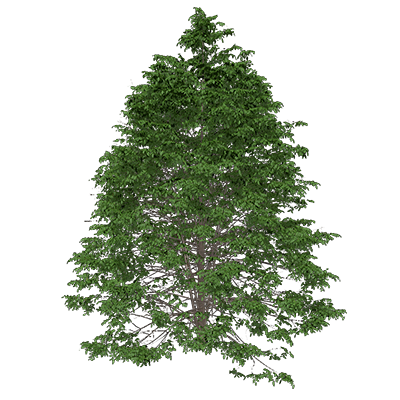
American Yellow Wood
More details →
More details →
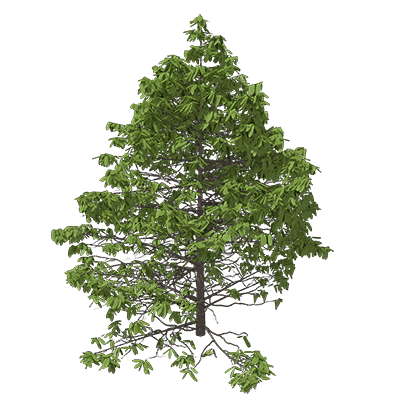
American Chestnut
More details →
More details →
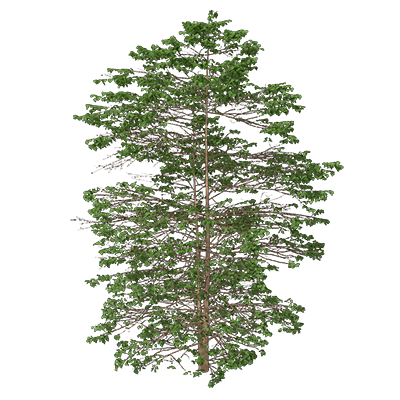
Washington Hawthorn
More details →
More details →
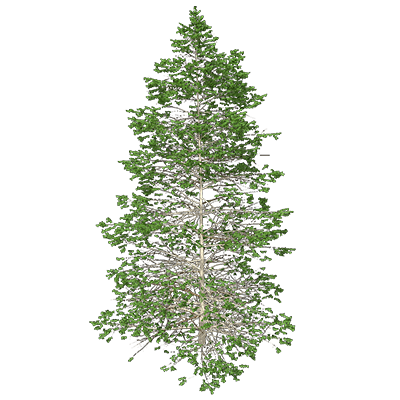
Paper Birch
More details →
More details →